Machine learning and remote sensing: New evaluation tools, especially to measure land-use
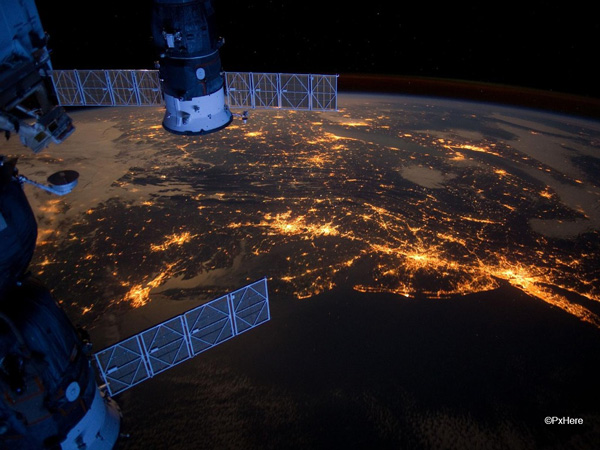
In August 2021, 3ie and New Light Technologies co-led a series of capacity-building workshops with 10 researchers from the African Population and Health Research Center on the potential to use remotely-sensed geospatial data for impact evaluations. This blog is the first in a series of four in which workshop participants reflect on the uses of remotely-sensed and geospatial data.
The use of Geographic Information System (GIS) technologies and remotely-sensed data allows us to map and quantify any changes that occur on a range of spatial outcomes, mainly by means of images collected from sensors onboard satellites. GIS technologies create new possibilities for better decision-making through a suite of components to improve measurement approaches. Remote sensing data and technologies allow researchers to collect and analyze information without on-the-ground work.
In Africa, the COVID-19 pandemic presented challenges for current data generation systems which are limited to face-to-face interactions. But technology offers new options to automate some functions in the data ecosystem, thus opening the possibility of using big data, including geospatial data, to understand and evaluate the impact of programs such as the current interventions to combat the COVID-19 pandemic. A large volume of data accumulated from satellites is freely-available, but there is still a dearth of information and analysis concerning Africa. Data from satellite imagery can provide information on land use and land cover, quantifying changes over time to inform the planning of interventions. Analytical techniques enable us to detect and measure trends in agricultural productivity and the amount of available recreation space, for example.
In the context of impact evaluations, geospatial data can allow the measurement of the outcome of an intervention. Artificial Intelligence (AI) and machine learning (ML) techniques are becoming an integral part of impact evaluations to develop classifications of data, such as with land cover and land use based on satellite imagery. The added value is that remote sensing data can be used for one part of an impact evaluation and then socio-economic data collected through traditional means can complement or validate it.
The African Population and Health Research Center (APHRC), International Initiative for Impact Evaluation (3ie), and New Light Technologies (NLT) are promoting the application of emerging impact evaluation techniques such as geospatial impact evaluations as well as innovative data-driven approaches such as big data analytics. All these can efficiently inform interventions in sub-Saharan Africa.
Source of Image: New Light Technologies Inc.
Artificial intelligence and machine learning help translate data into meaningful information that can be used for informed decision-making. These approaches are becoming increasingly important due to a rise in the quality and quantity of remote sensing data with higher spatial and spectral resolutions. Machine learning techniques can efficiently analyze the huge amount of data currently produced by modern Earth Observation (EO) satellite missions with the availability of high-performance computing platforms. Remotely-sensed data–earth observations collected by sensors on satellites, drones, or other sources– allows mapping and monitoring in real-time and across a broad region.
Changes in land use and land cover (LULCC) have been identified as key measures for studying ecological and environmental changes. LULCC information extracted from satellite images, as well as climate modeling and socioeconomic indicators, are critical in advancing interdisciplinary research. Our upcoming blogs this week on agricultural productivity and the urban expansion of Nairobi will highlight these opportunities. With remotely sensed measurements it is now possible to conduct both baseline mapping and subsequent monitoring, to identify the LULCC from year to year. This knowledge can support the development of strategies to balance conservation, conflicting uses, and developmental pressures. Land use studies are being driven by issues such as the removal or disturbance of productive land, urban expansion, and forest loss.
Traditional methods for gathering data are not adequate for multicomplex environmental studies, due to the complexity of multidisciplinary datasets. New technologies and data like those collected by satellite remote sensing and analyzed with Geographical Information Systems (GIS) provide new options to study and monitor the dynamics of natural resources for environmental management. Four examples of how artificial intelligence and machine learning are used in the field of remote sensing to map land-use are here (1), here (2), here (3), and here (4).
Applications for use in our work at APHRC
The public health response to COVID-19 requires a multidisciplinary approach, and focusing on a geographical location allows a holistic perspective that considers the interplay of human and biophysical. A lot remains unknown about the role of the environment on disease and its impact on decision-making in public health response. This situation offers the opportunity to combine data from disparate sources in developing predictive models using artificial intelligence and machine learning algorithms to inform public health decisions in Kenya and Malawi.
Our IDRC/SIDA Global South AL4COVID project is setting up a platform that integrates various data sources such as environmental and economic data with an AI actor model that takes into account the availability and quality of data sources over time. The system aims to inform risks of hospital admissions. We consider aggregated measures of environmental factors such as weather, pollution, and the prevalence of insects, then analyze how they can be associated with individuals and assimilated in the models for decision-making. This approach uses environmental data from Google satellites combined with clinical, behavioral, and socio-economic data to deepen our understanding of the impact of COVID-19. Another possible for application at APHRC is the use of remote sensing data from satellites on dust cover and air pollution in predictive models on the onset of dust storms and pollution. These models then inform public health responses on triggers for asthma and respiratory infections. Setting up a system for tracking the onset of those events through a web-based platform and timely alerts will assist health care providers in identifying the need for increased services for asthmatic patients and other patients at risk of respiratory infections within our Nairobi Urban Health Demographic Surveillance System in Nairobi.
These new approaches provide opportunities to better measure outcomes and evaluate the impacts of development interventions, allowing policymakers to accurately estimate the intended or unintended consequences of policy interventions.
For more on remote sensing in impact evaluations, check out 3ie's earlier blogs about three ways to start using remote sensing to measure impact, why big data will not be replacing surveys any time soon, and use of big data in the pandemic.
Add new comment